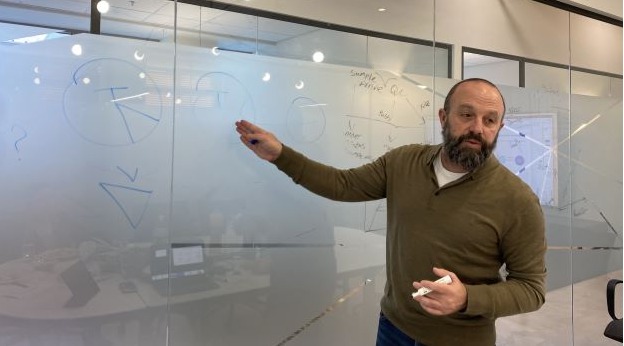
Ofer Sharon, M.D., CEO, OncoHost/company courtesy
Artificial intelligence and machine learning could change the face of cancer diagnostics.
Today, physicians may run blood tests to find biomarkers specific to cancer, conduct imaging tests via MRI, CT or ultrasound to visualize a potential tumor or biopsy to analyze tissue up close.
But these methods have drawbacks, including the possibility of not catching the cancer at all.
AI and ML can be given datasets to “learn” from, ultimately improving accuracy for detecting malignant cells.
Predictive models can use genetic information and other factors regarding a patient’s lifestyle to determine their likelihood of developing a certain type of cancer. This same information can also be used to identify how the cancer will respond to different treatments.
Some of the most difficult-to-treat cancers – including glioblastoma multiforme (GBM), ovarian cancer and non-small cell lung cancer (NSCLC) – could benefit from recent advances in AI/ML.
Helping to Predict Brain Tumor Progression
GBM remains one of the deadliest cancers, with a median survival time of 15 months and a five-year survival rate of only 5.5%, according to the National Institutes of Health.
Understanding the progression of GBM is challenging because the tumor must be treated quickly and aggressively for the best possible outcome. There is no time to wait and see how it will grow.
This leaves researchers at a loss for creating precision therapeutics. Without a roadmap of the cancer’s progression over time, it can be difficult to predict how a tumor might grow or the best time for surgery.
Researchers from the University of Waterloo and University of Toronto partnered with St. Michael’s Hospital to analyze MRI data from GBM patients. A unique dataset of five patients who chose not to have their tumors treated provided data that demonstrated how untreated GBM tumors grow over time.
The team developed a method that combined existing mathematical models with novel artificial intelligence approaches to predict patient-specific tumor progression.
“GBM tumors are super resistant to a lot of treatments. They’re super fast-growing and lead to a lot of recurrences after treatment,” Cameron Meaney, leader of the study and Ph.D. candidate in applied mathematics at the University of Waterloo, told BioSpace. “Projections of tumor progression that are patient-specific can be more accurate for answering questions about timing of treatment.”
With more data collection, Meaney said it’s possible physicians could utilize software that takes MRI images of a patient’s tumor and uses those images to predict future tumor growth.
Getting to this point will require collecting more data that shows how treatment affects tumor progression, Meaney said, and then using that data to make predictions specific to treatment.
Meaney added that ML models could one day help determine the most effective way to attack the tumor or identify optimization schemes to make treatment more efficient.
A New Approach to Ovarian Cancer Detection
Just as deadly, ovarian cancer is often labeled “the silent killer”.
Israeli femtech company Gina Life takes a different approach to the detection of ovarian cancer. Three-quarters of patients are diagnosed with metastatic cancer, and survival rates vary between 5% and 20%, Inbal Zafir-Lavie, Ph.D., co-founder and CEO, told BioSpace.
If detected early, however, she said survival rates are between 45 and 90%.
While most diagnostic approaches involve a blood test, Gina Life’s test utilizes vaginal secretions, a biofluid in the vicinity of the tumor. The test leverages a panel of unique, proprietary biomarkers supported by AI.
“The ovaries are small and they’re white, so for an early-stage, small tumor, the signal in the blood is low,” Zafir-Lavie said. “Basically, trying to detect early-stage ovarian cancer in the blood is like taking a blue eye drop and dropping it into a pool. You can barely see the signal.”
Gina Life applies machine learning in a system that learns what a healthy woman looks like.
“Now, we can add ovarian cancer patients and ask the system, where are the differences? With the human eye, this is really hard to do. I can see it, but I can’t see the complexities, and we’re looking for a fingerprint of biomarkers,” Zafir-Lavie said.
She emphasized that the company does not allow the machine to run wild.
“We’re always applying our domain knowledge…We’re always verifying that [the results] make sense.”
Optimizing the Power of Anti-PD 1/PD-L1 Therapies
AI/ML can play a vital role in directing treatment as well.
PD-1/PD-L1 inhibitors like Keytruda (pembrolizumab) and Opdivo (nivolumab) have been instrumental in treating non-small cell lung cancer.
These immunotherapies work by blocking the interaction between PD-L1, which is expressed on the cells of many tumors, and PD-1, which is highly expressed on many immune cells. They work for between 20 and 50% of patients. This poses a challenge for physicians.
“We don’t have good biomarkers for immunotherapy,” Ofer Sharon, M.D., CEO of OncoHost and chair of the Precision Oncology session at the upcoming Biomed Israel conference, told BioSpace. “A lot of the work by the clinician today is done based on clinical experience and sometimes trial and error.”
In stage 4 NSCLC, up to 80% of patients will be treated with immunotherapy – either monotherapy or in combination with chemotherapy. Currently, if the tumor expresses less than 50% PD-L1, a combination is recommended, while tumors expressing more than 50% PD-L1 should be treated with monotherapy or a combination, Sharon said.
There are currently hundreds of active trials involving various combinations with PD-1/PD-L1 inhibitors.
In a few years, “The decision tree for first line therapy in lung cancer alone will involve numerous choices,” Sharon said.
So, OncoHost developed the PROphet platform, which can help physicians understand the interplay between the tumor, the patient and the therapy. With a single blood test, PROphet analyzes proteomic patterns with AI algorithms to predict an individual patient’s response to anti-PD-1/PD-L1 therapies – so far, very accurately.
PROphet attempts to answer the following questions:
1. What is the optimal first-line therapy?
2. What drives the tumor’s resistance?
3. What are the next steps?
A study published in the Journal for Immunotherapy of Cancer in June 2022 highlighted a predictive proteomic signature revealed by the PROphet platform for analyzing treatment resistance in NSCLC. OncoHost launched the PROphet NSCLC test in the U.S. in February.